.avif)
No one-size-fits-all: The many levels to getting the sample size just right
Let's try something: google 'sample size' – what did you get? One of the first search suggestions that come up is definitely a 'sample size calculator'. But let's be honest, how applicable is it for real-life research needs?
To oversimplify, generally, the bigger the sample size, the better – more respondents, more answers, more predictive insights. But is that move always business savvy?
Let's take a look at some cases where being smarter and creative adds value when picking the right sample size:
On your mark, get set, go psychometric
Ask any researcher, and they will tell you that the guiding light to picking the right sample size is choosing the margin of error (be it 99%, 95%, or 90% - in commercial research, 90% is typical, while in academics, 95% and 99% are the usual go-to's). Typically, a larger sample size means a smaller error if all other variables are stable.
Following the basic psychometric principles, you would opt to have (at least) 5 times more respondents than the number of items. While traditionally, the item is a survey question, it can also be the number of SKUs on a virtual shelf. For instance, if you have 100 SKUs - the rule of thumb is to have 500 respondents.
But for shelf studies, even 300 may be enough when researching the impact on brand and category, which is most often the case. With more respondents, valid and robust insights could be made on an even more granular level of analysis.
Not every glove will fit the different methods
Of course, apart from the margin of error, the sample size also depends on the methodology used. While some surveys can include a minimum of 50-75 respondents, Virtual Shopping exercises with a standard-sized shelf need as many as 300 to collect accurate results. To further prove this, a standard (before cleaning the sample) for Facial Coding is 80, while Eye Tracking requires 60 and still yields highly predictive insights.
Why the vastly different numbers? Well, both Facial Coding and Eye Tracking provide results that are universal for humans and highly biologically determined. This means that we can use smaller samples to determine the way that people perceive specific stimuli. In other words, adding more respondents to the study will not significantly change the results, nor will it enhance the results.*
Moreover, methods such as RTM, MaxDiff, Conjoint, Findability, etc., require a certain number of people to fully complete the exercise as a minimum to get the insights we need, which can change the starting sample size.
It is also essential to keep in mind the analyses that need to be done so that the final output is robust enough at the most granular level of analysis required for the initiative. For example, as our Shopper Insights Director Dobrinka Vicentijevic explained - if you are doing segmentation and want to get 4 segments of consumers with different characteristics, you would start from the group you expect to be least represented and build the sample size from there. If the smallest group you expect to analyze is represented by around 10%, then you will need 1000 people to get 100 of them – and the same logic can be applied to other methods, starting from their minimum sample for the subgroup.
However, Milica Kovac, EyeSee's Senior Product Manager, underlines the importance of not having too many quotas and cross-quotas in online studies - it requires real-time monitoring of each and every respondent that comes into the database and knowing exactly which quotas will close when. An alternative for some studies conducted online would be a carefully planned combination of softer quotas and statistical procedures that follow. But what should you do if you need to conduct a specific analysis (e.g., brand split) but don't have enough users?
What Heather Graham, Client Service Director at EyeSee, believes is a solution for this is knowing the brand user volume market share. When it comes to testing pack redesigns, for example, this allows us to estimate the natural fallout of users from a category-representative sample and determine just how many are needed to boost the sample. This further ensures an analyzable base size of users for pack redesigns.
Yeah, statistics are cool, but do you know your consumer?
But then there are times when the sample size is not dictated just by the analysis - but by the end-user of the study results. For example, in category management studies (planogram and decision tree studies), the main KPI is sales potential.
So, when the client knows that 1% of real sales growth brings millions in revenue to the company, showing that 15% in sales growth is statistically irrelevant due to a small sample size is simply not the way to go. That's why the sample is increased for the sake of usability and reliability of the obtained data for such studies.
However, if the incidence rate of a particular consumer group is extremely low - instead of increasing the sample drastically to naturally capture enough consumers from that group, an adequate sample is taken for all other required target groups instead. Then the number of people with extremely low IR is boosted as a separate sample.
Be savvy when choosing the right research design
A monadic research design entails a study in which the respondent evaluates only one product, concept or ad - rather than comparing it to different test stimuli. In doing so, you can ensure that the data you get is not influenced by any other stimuli.
But there is a good reason why sequential (monadic) designs also exist, and the insights they unlock can be priceless when compared to the standard monadic approach – especially when testing the sales potential of new products. Not only does it require a smaller sample, but by having the same people make two shopping trips, one in a competitive environment before the new product introduction and then another one with the new product launched and implemented on the shelves or webpages, it captures the all-important switching behavior and potential cannibalization effects.
Why are we talking about the design so much? Well, sample sizes are a part of the research design and having a comprehensive understanding of the design characteristics opens up room for sample size optimization!
Key takeaways:
- Don't throw psychometric principles out the window - but leave space for optimization and sample tinkering to boost the reliability of research findings
- Different methods have their own rulebook –their requirements can be vastly different, so it is vital to have an in-depth understanding of each one
- Know who your audience is – both in terms of who will use the data and the target audience you recruit
- Be picky about research design - it is not impossible to answer more questions with a seemingly smaller sample size
*For a more detailed breakdown of choosing a sample size for these methods, check out this Proof of Method!
Nestle Purina x EyeSee
@Quirk's Innovation in the Inflation Era webinar
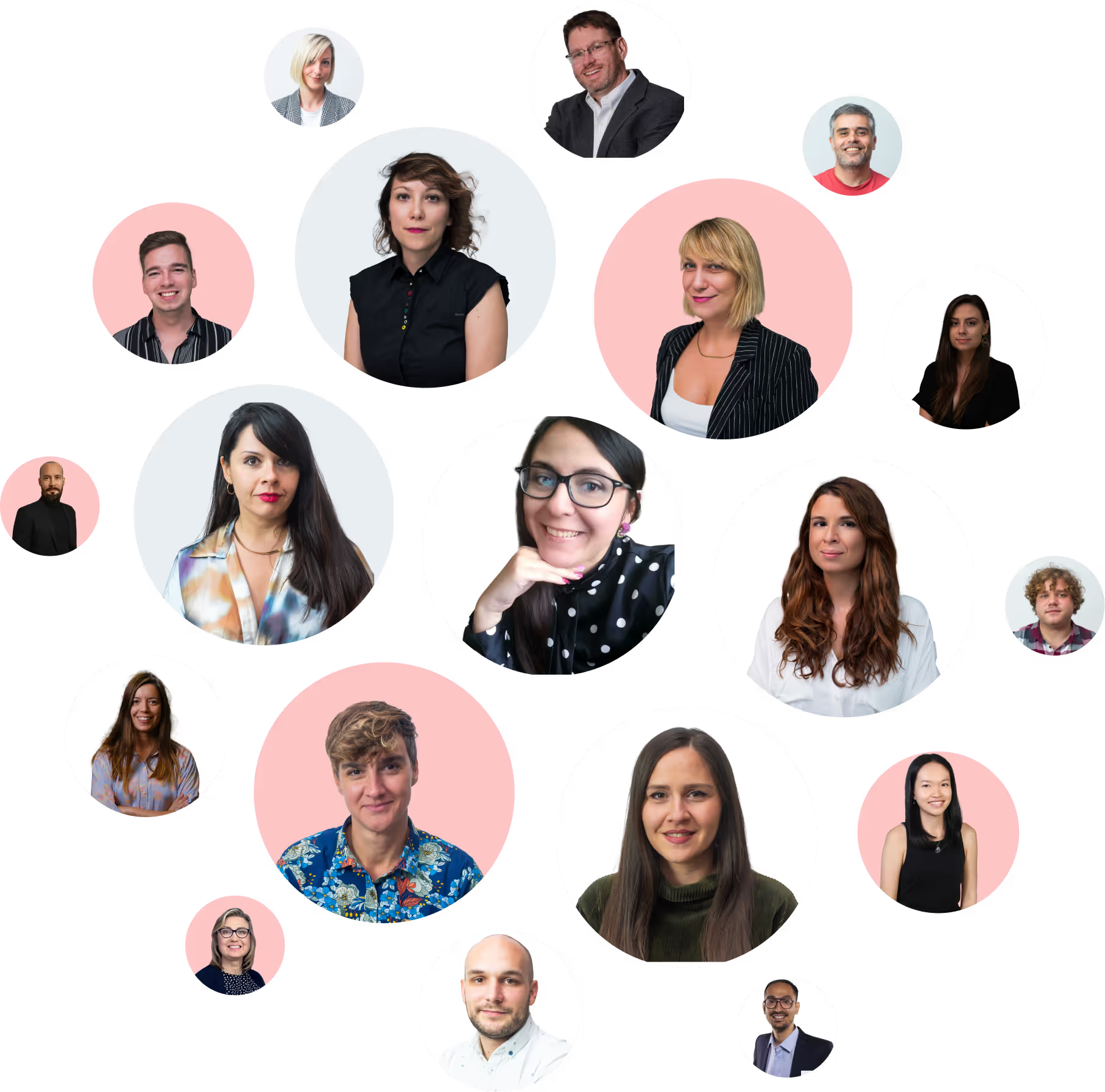