
A behavioral bypass of the Qual vs. Quant debate
Too often, companies conduct qualitative studies when quantitative studies were necessary in their place. This happens because people are biased towards stories. The proponents of qual will say vice versa.
Nevertheless, both qual and quant are valuable and complementary research methodologies – only if we understand their (dis)advantages, when to use and when to avoid them and utilize them for specific research objectives.
A behavioral perspective on qual – the problem with stories
From a high-level perspective, qual is conducted to explore and build stories, and quant for the confirmation and validation of your hypotheses. Supporters of quantitative research are prone to see qualitative as less relevant and not representative because of its small sample sizes. Moreover, it is perceived as less-than-objective due to the possible bias of the researcher guiding the discussion.
Dan Ariely makes some interesting points about qualitative research. “Research in psychology and behavioral economics has shown time after time that people have bad intuitions. We are very good at explaining our behavior (sometimes shocking and irrational), and to do so we create neatly packaged stories – stories that may be amusing or provocative, but often have little to do with the real causes of our behaviors. Our actions are often guided by the primitive inner parts of our brain – parts that we can’t consciously access — and because of that we don’t always know why we behave in the ways we do; still, we can compensate for this lack of information by writing our own versions. Our highly sophisticated prefrontal cortex (only recently developed, by evolutionary standards) takes the reigns and paints a perfect picture to explain what we don’t know. Why did you buy that brand of fabric softener? Of course, because you love the way it makes your clothes smell like a springtime breeze when you pull them out of the warm dryer.”
The problem with that is – humans love stories and use them to explain things and decisions we don’t understand. After making decisions, we are prone to post-rationalizing why we made them and fill in the missing gaps in our perception of our behavior. As Ariely notes in his blog, we are all biased towards qual – he continues: “I suspect that the story here is linked to another one of our irrationalities: As human beings, we have an insatiable need for a story. We love a vivid picture, a penetrating example, an anecdote that will stay in our memories. […] A single example feels real, where numbers are cold and sterile.”
Is traditional Quant error-free?
On the other hand, in the qualitative circles, quantitative research methodology is criticized because its hard data and numbers are too general and miss out on deeper insights without a human touch. However, analyzing surveys is not always straightforward – e.g., someone can select a 4 out of a 7 point Likert scale, and someone who is more pessimistic might choose a 3 but in reality have the same opinion on the topic in question.
Experimental research design and behavioral methods can help with this. For example, you can test 2 variations of package design in a virtual shopping environment. Insights gained this way (e.g., design B is bought by 25% more shoppers than design A) is much easier to understand and act on than a claim stating that 10% more shoppers scored design B higher in the top 2 boxes of a 7-point Likert scale question. Most importantly, you can identify the impact as you can compare the sales (penetration, market share). It is crucial to understand what you can test successfully and the things that surveys can not verify.
Quant, Qual and beyond
For growth spurred by insights, we need both exploration and validation as guiding principles. Having their variability in mind, both approaches should be employed with care. Trust in qual only as far as it facilitates exploration, and leave the validation to behavioral quant experiments. Behavioral research can bypass the very human biases we face in the minds of the respondents and the moderator likewise. It gives you the ability to compare several solutions to determine which one performs best and how it will impact your sales, quickly, with a large sample, with high predictability – by relying on implicit consumer behavior.
Nestle Purina x EyeSee
@Quirk's Innovation in the Inflation Era webinar
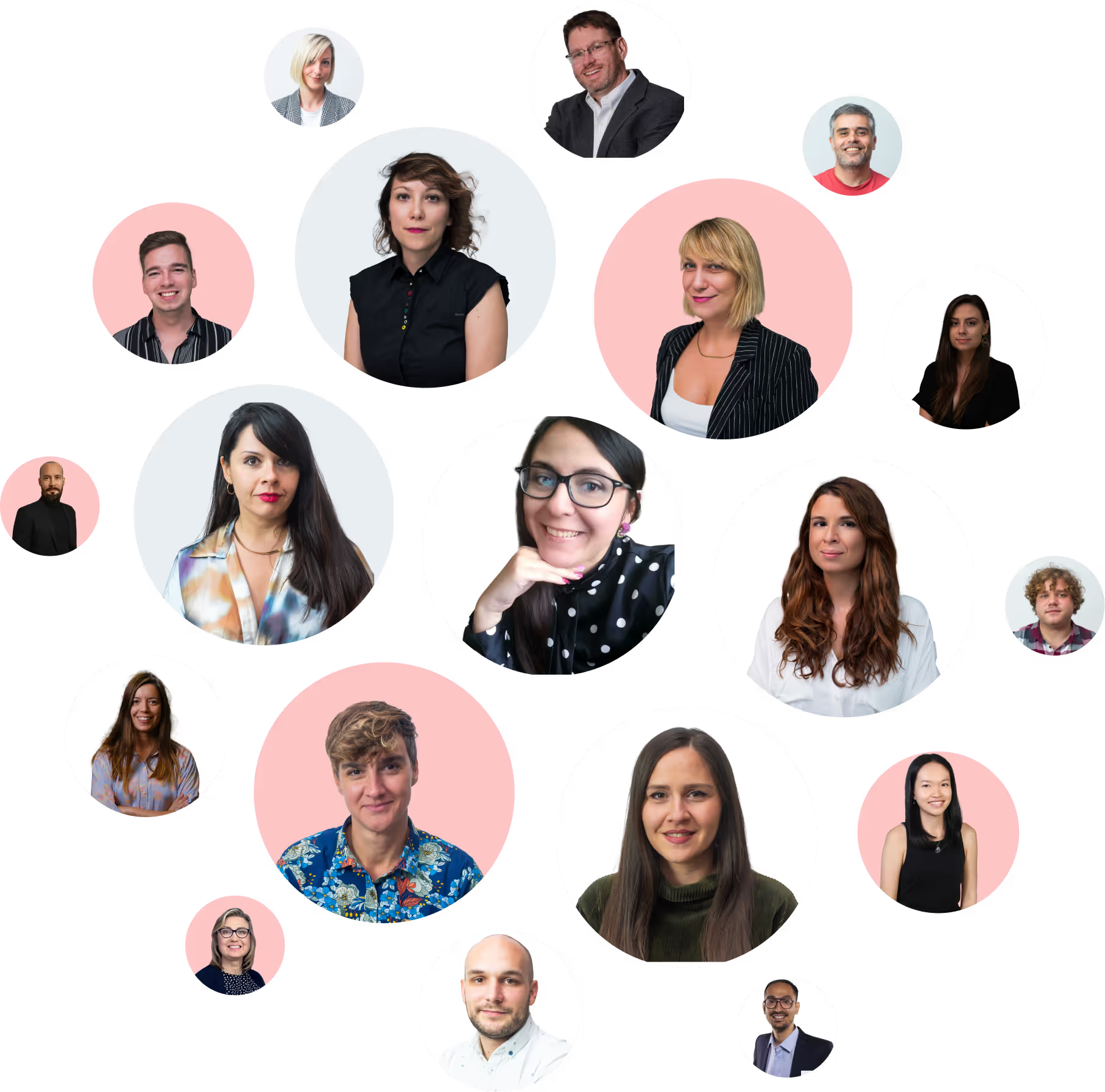