
All that buzz: How to use AI in consumer research
Despite the ever-increasing and all-encompassing ‘buzz’ of AI, just like many other topics (machine learning, BeSci) it has been around for at least 50 years. However, the pandemic has exponentially accelerated the need for change and escalated the willingness to adopt new tech. The question is – does using AI in consumer research add value, and how and when to employ it so that it does?
What is the scope of AI in consumer research?
The promise of AI is excellent; at EyeSee, most of our fundamental methods use some form of AI (webcam-based eye tracking, facial coding, analyzing virtual shopping data, etc.). However, AI and machine learning are not one-solution-fits-all. It is essential to understand the scope (width) and the limitations (depth) of AI.
Width of AI: AI and machine learning can be used for many applications in market research: (1) collecting data by replacing current methods, process optimization (automation, DIY…), analyzing datasets in a way that was not possible before (e.g., big data)… AI is a means to an end and should never be the goal. Take a step back and ask yourself: What am I trying to accomplish with using AI?
Depth of AI: The limitations need to be taken into account. AI is only as good as the data we feed it – with the economic crisis already disrupting our lives and consumer habits, be wise and carefully estimate its power of foresight, especially when it comes to predictive models based on (past) data. Another thing to be mindful of is checking the noise in the dataset and the error margin. Let’s take a look at eye-tracking. Right now on the market, there are AI-based solutions that predict the gaze direction. Oftentimes, a 5-10% increase in visibility for packaging is significant and can make a huge difference – but these solutions have error margins much bigger than that. The second question presents itself: Is AI (or any other hot buzz) the best solution for my current problem? Depending on what you need, the answer might be different. For example, early screening of new concepts can be done using AI-based methodologies, but if you need validation, a superior choice would be experiment-based solutions using real shoppers, such as virtual shopping.
In many cases, a combined approach of doing research the way you are currently doing (e.g. experimental) with AI models might be the best way to move forward.
So what are the benefits of using AI solutions in research?
When done well, AI (or any other new technology buzzing around the industry) can create many advantages: impacting speed, time and costs, enabling access to new data, decreasing mistakes… But just like most decisions, you need to do a more in-depth analysis to assess whether it will fit your goal and is the best solution. You might learn that often a hybrid model is the best for covering all phases of your product development: e.g. using AI-based exploration and experiment-based validation.
What now – how do you approach implementing new tech in research?
There are several questions to answer before adopting any new technology in your studies:
- What are your objectives, and will they be solved with the new buzz? If yes, fantastic! Still, think under which circumstances it will add value. Testing a pricey SuperBowl ad with a DIY platform is probably not the best idea.
- Do you have the implementation skills to take up this new technology? How about the research skills? For most technologies, it takes 3-7 similar projects to take full advantage of the new tech. Agencies play a crucial role in diffusing innovations. They execute similar projects for many different clients – thus, their learning is steeper and experience broader.
- Is this your first project and you are not sure where to start? Be smart and pick the level of the study which will provide you with quick wins that demonstrate the impact of the new approach to your stakeholders. Often, the bottom-up approach – start with tactical optimizations and then address strategy. It sounds counterintuitive, but it might be the best.
- Do you have an efficient framework for disseminating new findings? A vital step final step is finding a way to socialize the latest insights – or approaches – across the organization. However cool your new tool is, if you don’t have broad support and buy-in for it, and a clear understanding of the new value or type of data gained, you will waste precious energy.
Kellanova x EyeSee
@Quirk's Pack Redesign webinar on demand
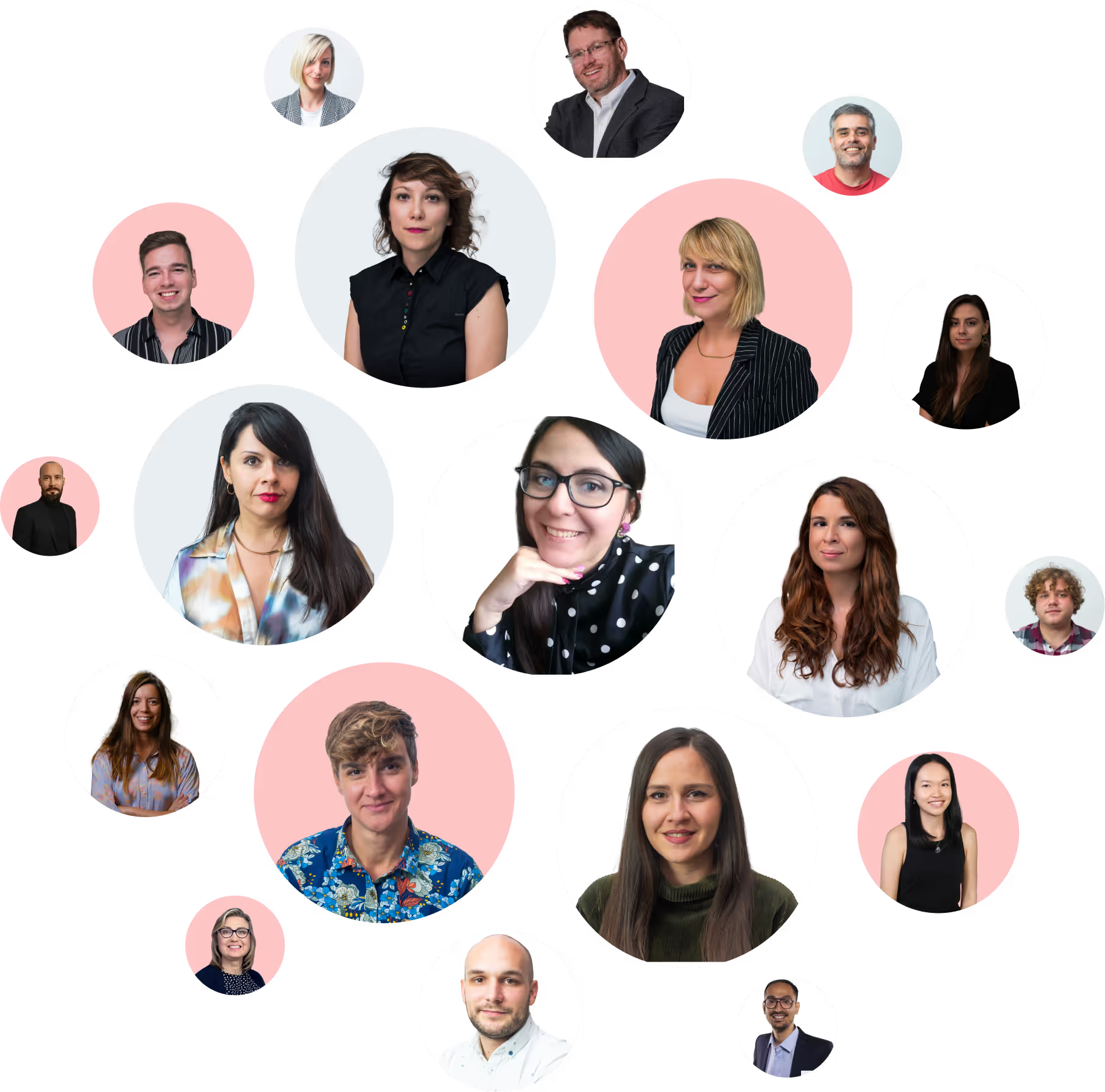